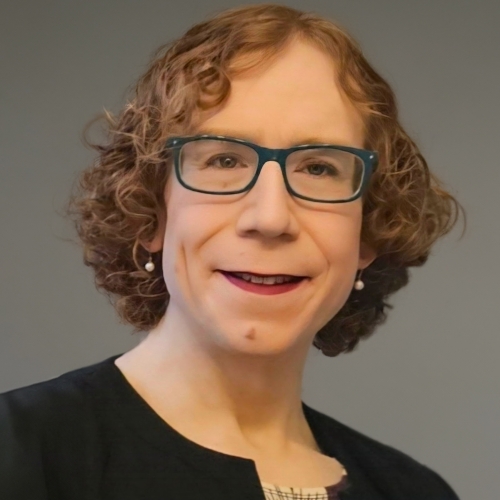
Ann Arbor
MI, 48109-0600 United States
Available to mentor
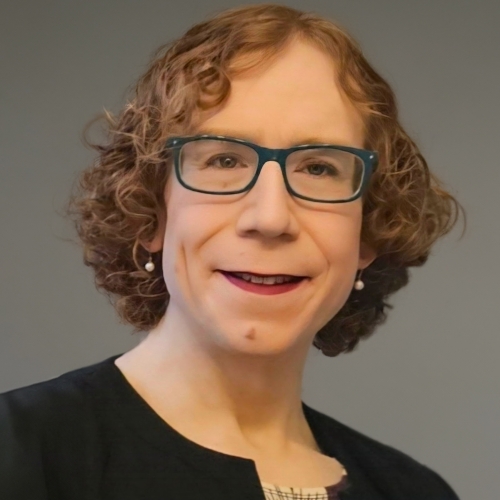
The regulatory networks of bacteria play a key role in their information processing capabilities, coordinating and executing interactions with their environments. Quantitative, predictive models of these networks would be tremendously beneficial for facilitating the development of new antimicrobial therapies, enabling synthetic biology applications, and understanding bacterial evolution and ecology. Ultimately, the aim of my laboratory is to build a multiscale framework enabling modeling of bacterial regulatory networks at any level of detail, from atomistic to cellular. To this end, we develop and apply high-throughput experimental methods for measuring biomolecular interactions and cellular regulatory states in vivo, and for profiling the phenotypic consequences of regulatory changes. In tandem with these experimental approaches, we use molecular simulation and mathematical modeling to obtain high-resolution insight into the biomolecular interactions driving regulatory networks, and the systems-level effects of altering them.
Freddolino Lab
-
Postdoctoral fellowColumbia University, Systems Biology, 2014
-
Postdoctoral researcherPrinceton University, Molecular Biology, 2011
-
PhDUniversity of Illinois at Urbana-Champaign, Urbana, 2009
-
BSCalifornia Institute of Technology, Pasadena, 2004
* Interplay of protein occupancy, chromosomal structure, and gene regulation in bacteria
* New mechanisms of bacterial transcriptional regulation
* High-performance methods for protein structure prediction
* Functions and physiological roles of poorly annotated proteins
-
Chen Z, Seman M, Fyodorova Y, Farhat A, Ames A, Levashkevich A, Biswas S, Huang F, Freddolino L, Biteen JS, Ragunathan K. Nucleic Acids Res, 2024 Oct 14; 52 (18): 10731 - 10746.Journal ArticleTracking live-cell single-molecule dynamics enables measurements of heterochromatin-associated protein-protein interactions.
DOI:10.1093/nar/gkae692 PMID: 39142658 -
Rakibova Y, Dunham DT, Seed KD, Freddolino L. mSphere, 2024 Jul 30; 9 (7): e0001124Journal ArticleNucleoid-associated proteins shape the global protein occupancy and transcriptional landscape of a clinical isolate of Vibrio cholerae.
DOI:10.1128/msphere.00011-24 PMID: 38920383 -
Zhang C, Freddolino L. Brief Bioinform, 2024 May 23; 25 (4):Journal ArticleA large-scale assessment of sequence database search tools for homology-based protein function prediction.
DOI:10.1093/bib/bbae349 PMID: 39038936 -
Zhang C, Freddolino L. PLoS Biol, 2024 Jul; 22 (7): e3002476Journal ArticleFURNA: A database for functional annotations of RNA structures.
DOI:10.1371/journal.pbio.3002476 PMID: 39074139 -
Haugen RJ, Barnier C, Elrod ND, Luo H, Jensen MK, Ji P, Smibert CA, Lipshitz HD, Wagner EJ, Freddolino PL, Goldstrohm AC. RNA, 2024 Jun 17; 30 (7): 866 - 890.Journal ArticleRegulation of the Drosophila transcriptome by Pumilio and the CCR4-NOT deadenylase complex.
DOI:10.1261/rna.079813.123 PMID: 38627019 -
Royzenblat SK, Freddolino L. EcoSal Plus, 2024 Jun 12; eesp00012022Journal ArticleSpatio-temporal organization of the E. coli chromosome from base to cellular length scales.
DOI:10.1128/ecosalplus.esp-0001-2022 PMID: 38864557 -
Zhang Z, Cai Y, Zhang B, Zheng W, Freddolino L, Zhang G, Zhou X. Brief Bioinform, 2024 Jan 22; 25 (2):Journal ArticleDEMO-EM2: assembling protein complex structures from cryo-EM maps through intertwined chain and domain fitting.
DOI:10.1093/bib/bbae113 PMID: 38517699 -
Zheng W, Wuyun Q, Li Y, Zhang C, Freddolino PL, Zhang Y. Nat Methods, 2024 Feb; 21 (2): 279 - 289.Journal ArticleImproving deep learning protein monomer and complex structure prediction using DeepMSA2 with huge metagenomics data.
DOI:10.1038/s41592-023-02130-4 PMID: 38167654