In 2022–2023, eight new faculty joined DCMB. Welcome!
Professor Kin Fai Au, starting in Winter 2023
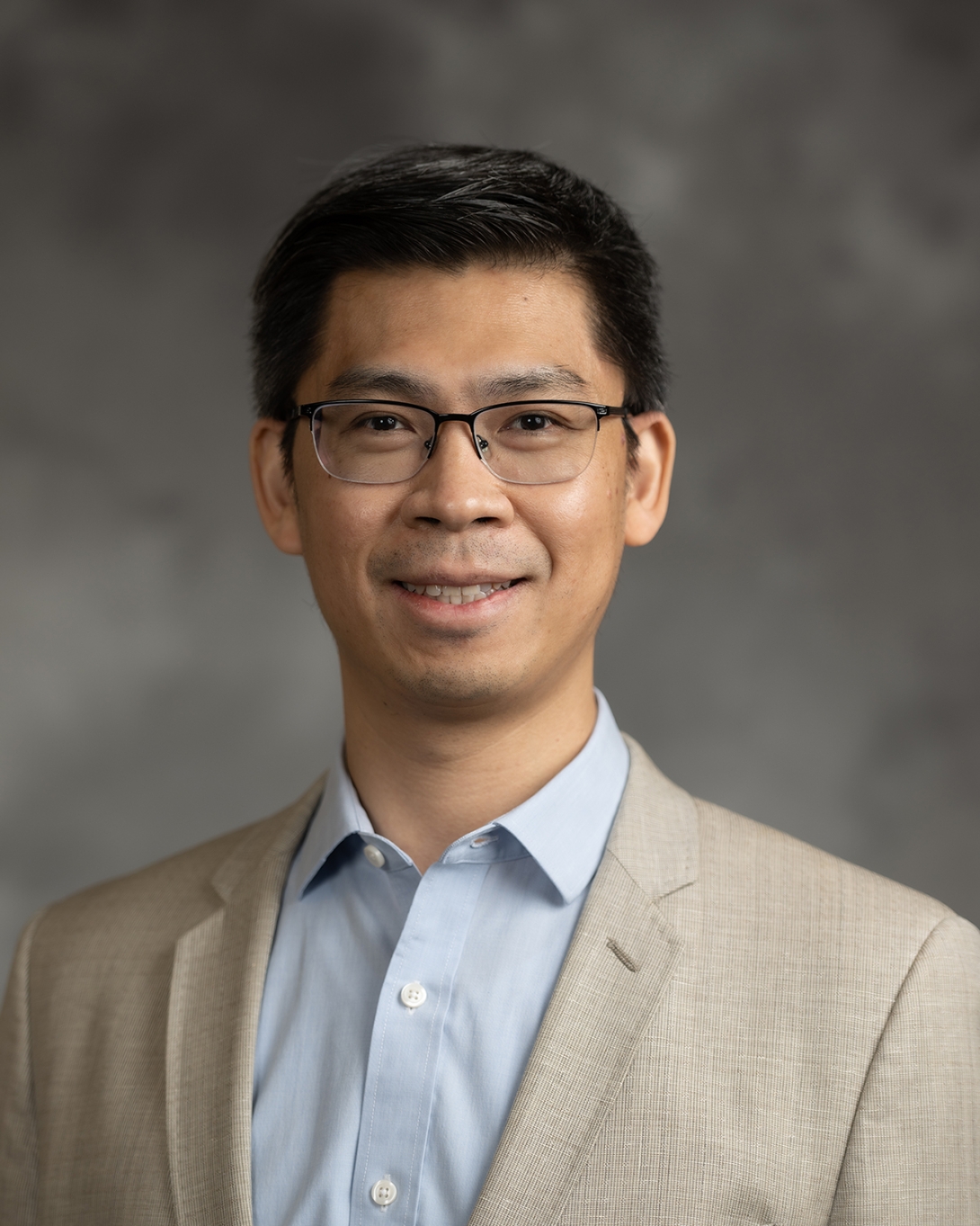
Dr. Au is currently an Associate Professor of Biomedical Informatics at Ohio State University. At DCMB, he will develop novel statistical and computational methods for analyzing high-throughput biological data, including but not limited to transcriptome- and epigenetics-sequencing data. His lab will also perform world-leading science in stem cell biology, developmental biology and cancer research using cutting-edge sequencing techniques from PacBio and Oxford Nanopore Technologies. One of his current projects aims to design advanced algorithms and statistical models for analyzing transcriptome data generated from third-generation sequencing platforms. These methods will then be applied to study the complexity of breast cancer and stem cell transcriptomes.
Assistant Professor Anne Draelos, starting in Spring 2023
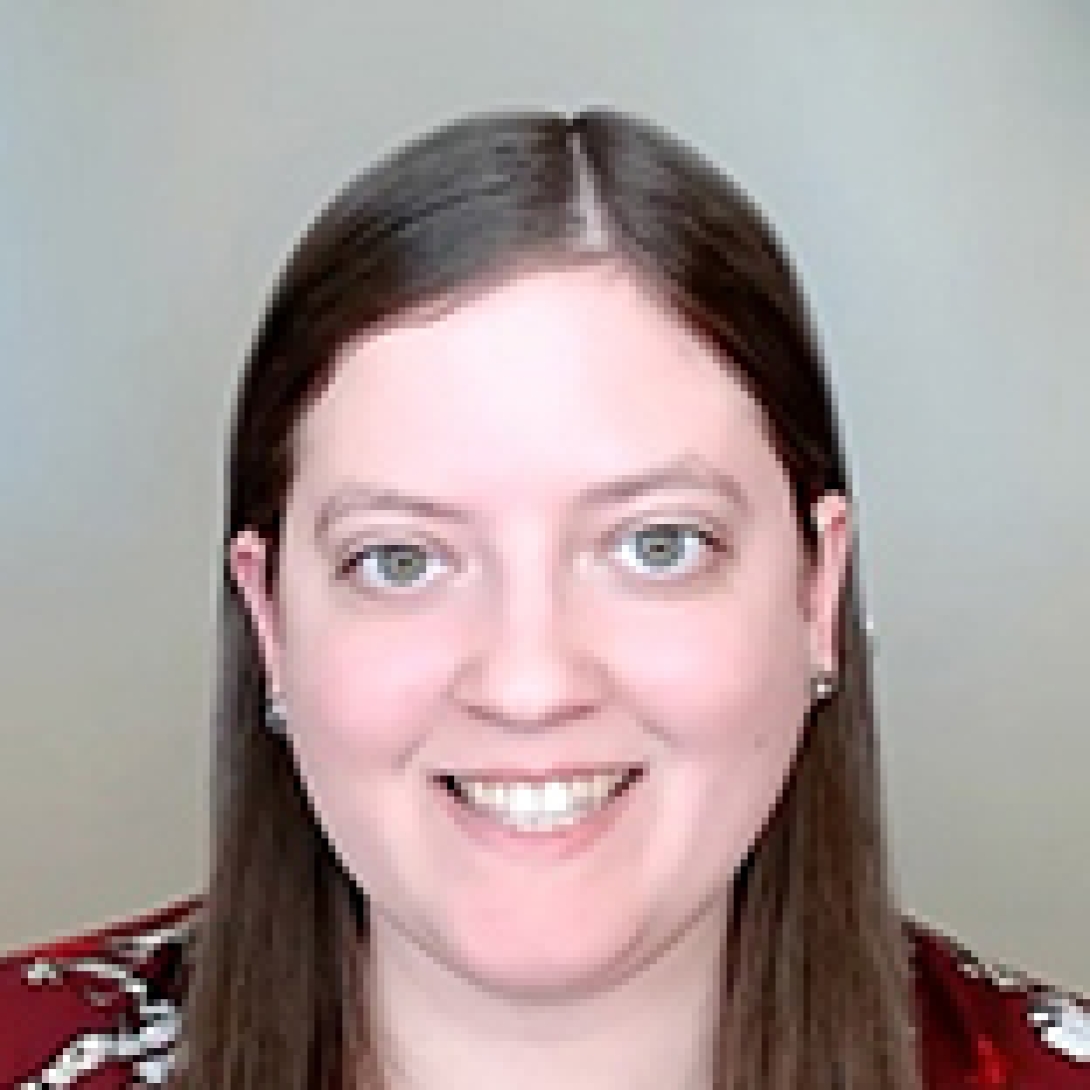
Dr. Draelos is currently a Swartz Foundation Fellow for Theory in Neuroscience in the Pearson Lab, Department of Biostatistics and Bioinformatics at Duke University. She will have a joint appointment in Biomedical Engineering Department (BME) primary (75%) and DCMB (25%). Dr. Draelos studies machine learning and statistical techniques to facilitate real-time analysis of neural data. She is broadly interested in the design and construction of new measurement and analysis methods. She has strong interests in adaptive experimental design, functional brain mapping, and linking neural and behavioral spaces through adaptive stimulation.
Assistant Professor Minji Kim, starting in Winter 2023
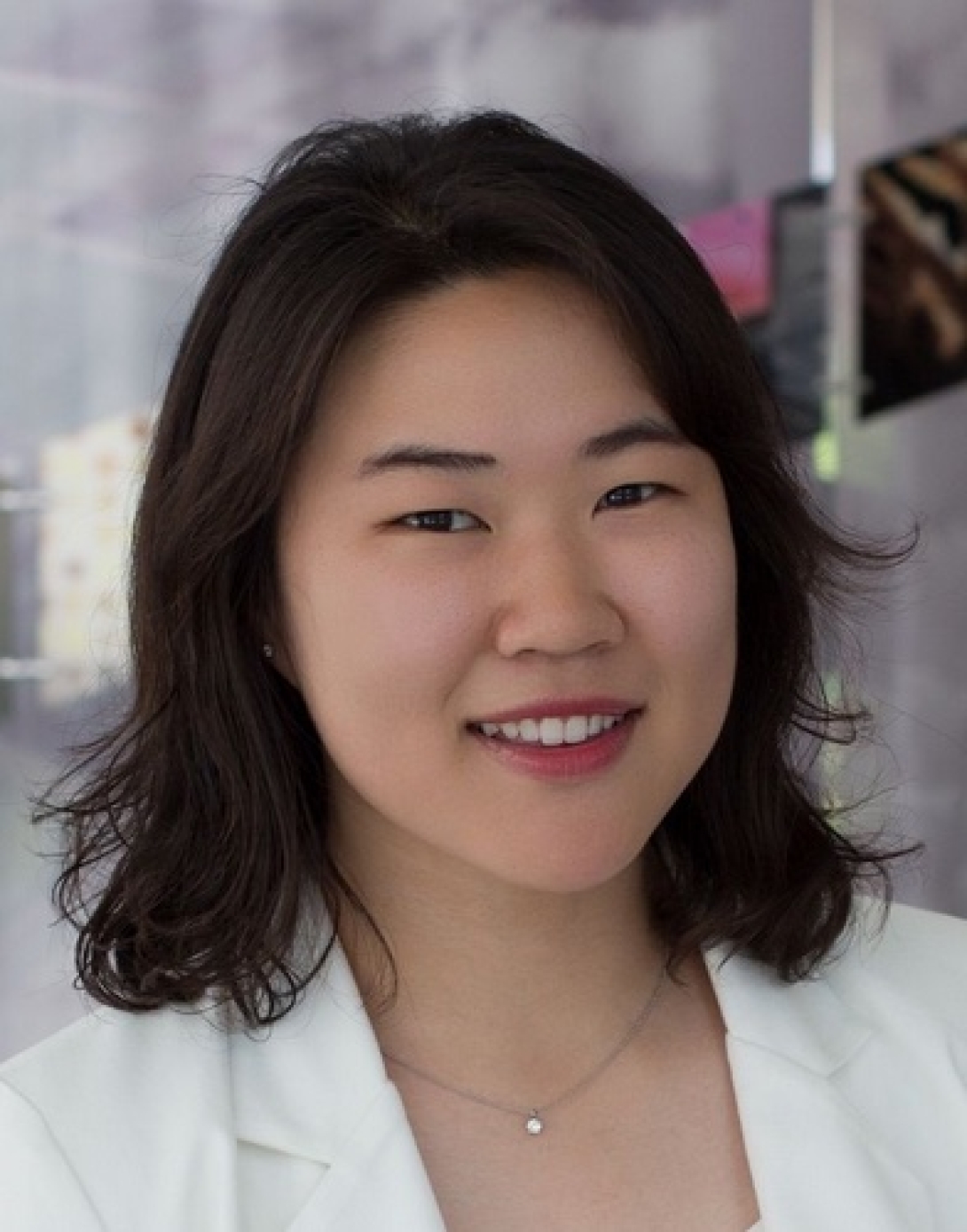
Dr. Minji Kim leverages 3D genome mapping technologies to investigate the role of chromatin interactions in gene regulations. With the advent of high-throughput sequencing and single-cell genomic techniques, researchers can now infer how our genome is organized inside the tiny nucleus—with a single-cell and single-molecule resolution. But the relationship between these genome structures and gene expression is still not clear. For example, it is known that changes in transcriptional landscape accompany differentiation processes in early development, yet the precision mechanisms controlling these transcriptional changes remain elusive. By applying 3D genome mapping technologies, Kim will identify which of the chromatin interactions are correlated with gene expression and potentially “instruct” cells to become a specific cell type. She will also develop computational tools and algorithms.
Assistant Professor Matthew O'Meara, starting Spring 2023
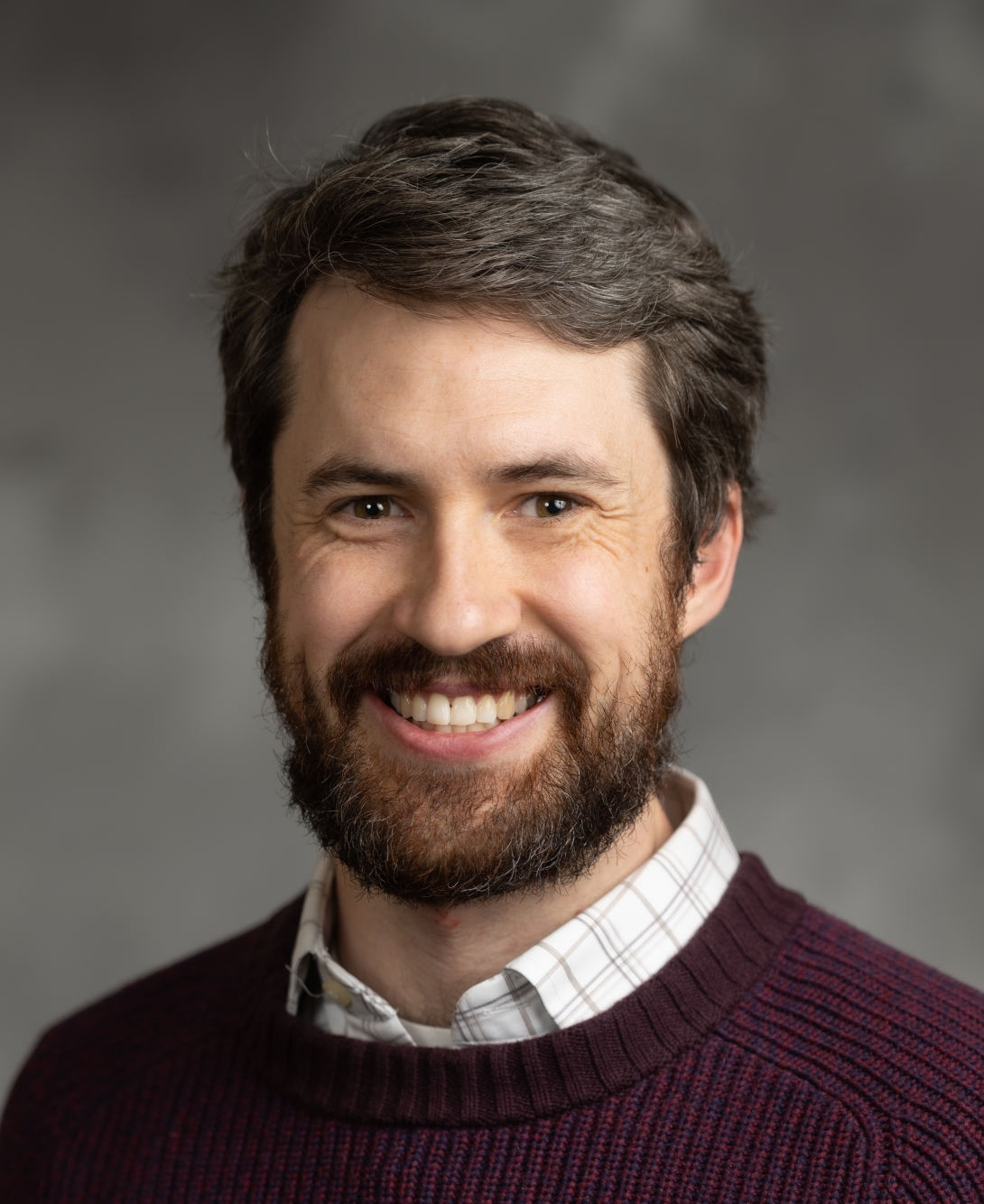
O'Meara's recent interests are in developing statistical and machine learning methods to model complex experimental design problems for pharmacology and biochemistry. Recent breakthroughs in experimental methods and rich simulation based models in the biological sciences promise to enable new insights and discoveries. However, as these methods scale, an increasing bottleneck is expert synthesis to plan highly informative experiments. By modeling experiments probabilistically, it is possible to holistically capture the assumptions and data, the decisions, and the outcomes. This rigor enables navigating the space of experimental designs to maximize the information gain and making new discoveries. Excitingly, recent breakthroughs in statistics and machine learning for building and fitting structured probabilistic models make it now practical to apply these models at scale.
Assistant Professor Meng Wang, starting Fall 2023 at DCMB and at the Institute for Heart and Brain Health (IHBH)
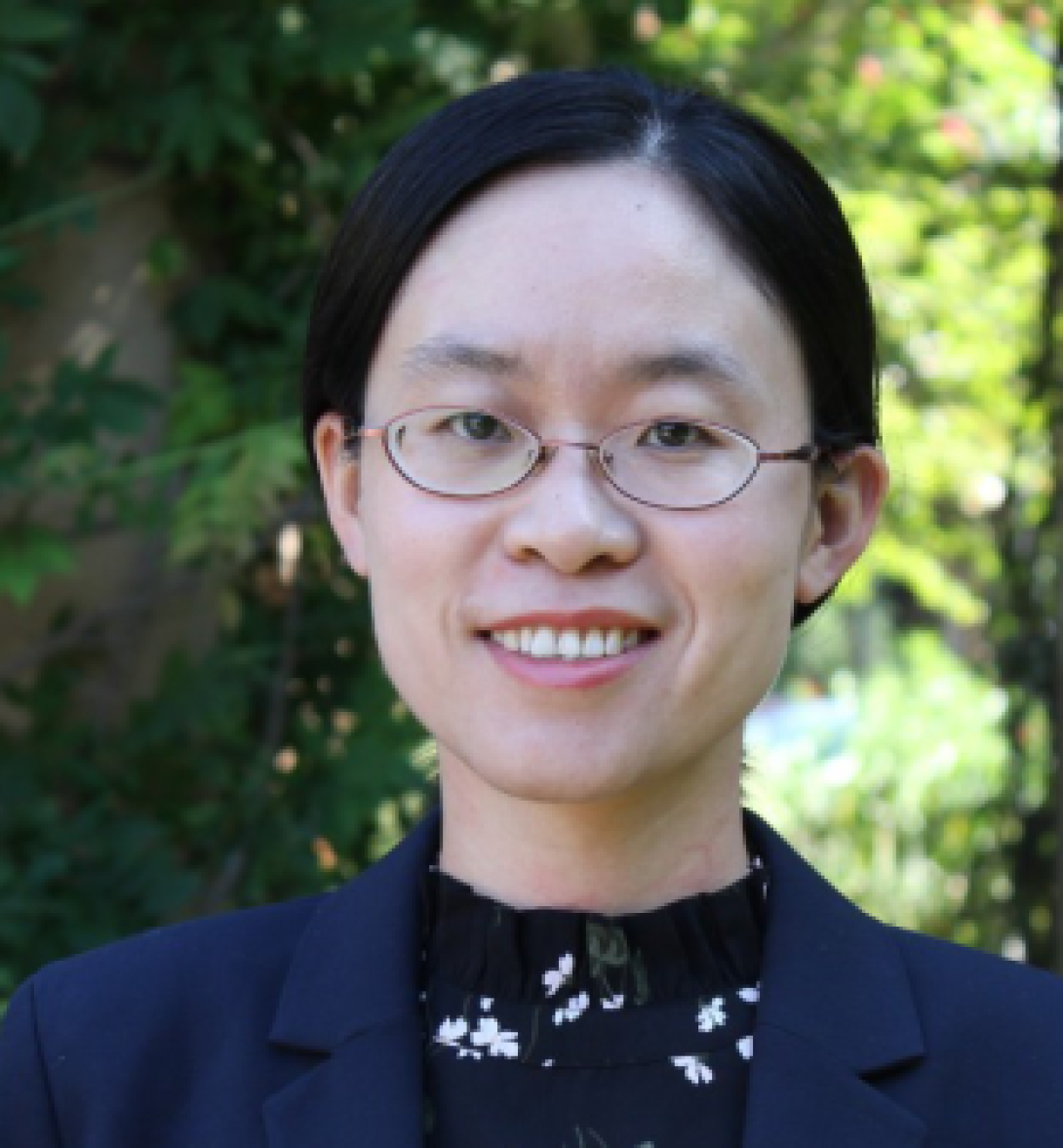
Dr. Wang’s primary research interest is to better understand the complexity of human biology and disease via multi-modal biomedical data integration. In this, her goal is to develop innovative statistical and computational methodologies to integrate multi-omics data, medical images, clinical measurements, and data from wearable devices, and to apply these methods to the early detection, intervention, and prevention of diseases.
At IHBH, Dr. Wang will work on developments of new methodologies to study multi-tissue responses to physical exercises and under disease models, especially the heart and brain.
Research Investigator Yunhao Wang, starting Fall 2023 in the Kin Fai Au Lab
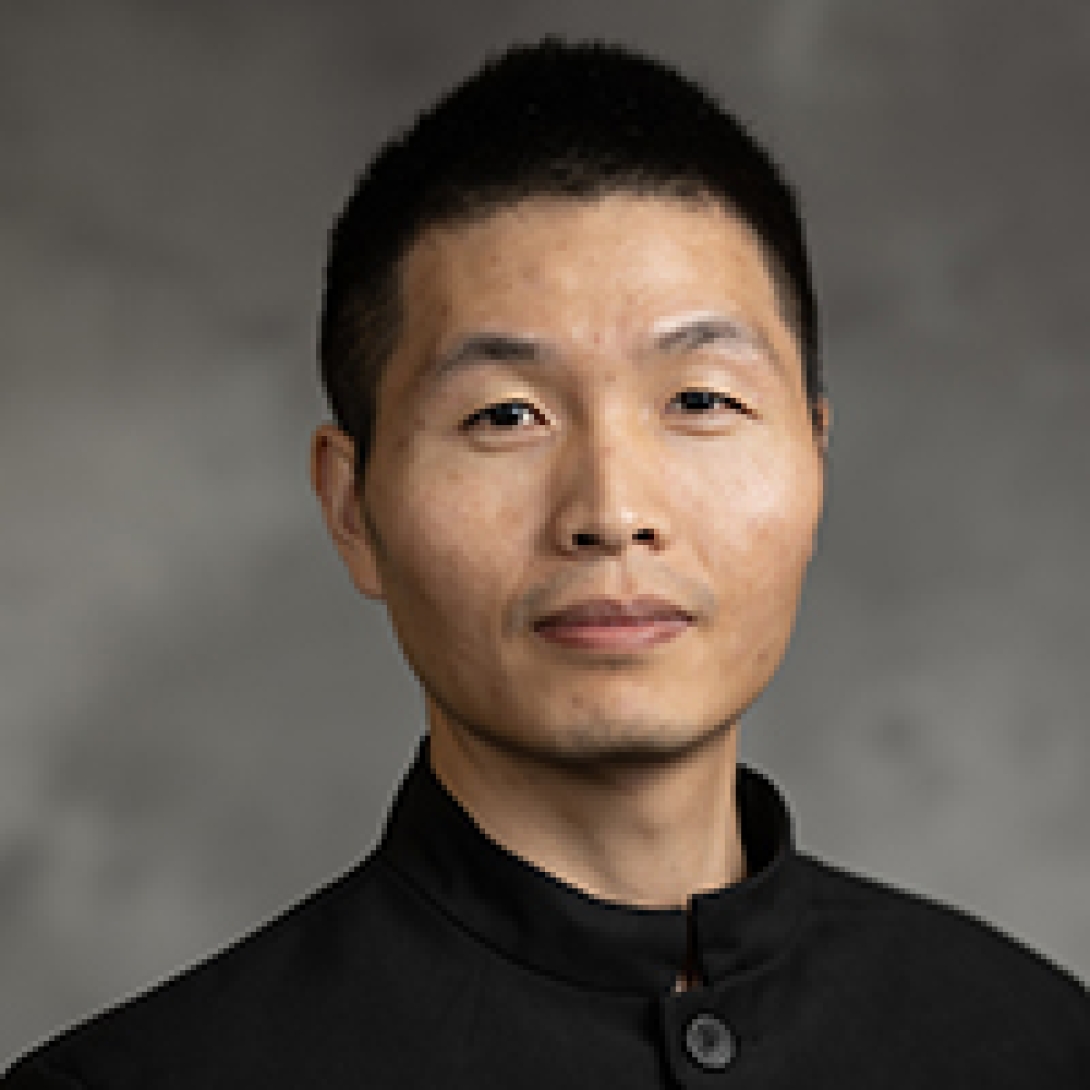
Dr. Wang develops both experimental approaches and bioinformatics tools to bridge a methodological gap between sequencing technology and biological applications. He explores the regulatory principles of gene expression and transposable element activation at multiple omics layers (i.e., genome, epigenome, transcriptome and epitranscriptome).
His area of expertise is in the delivery of novel biological insights through leveraging the advances of the cutting-edge third-generation single-molecule long-read sequencing techniques developed by Pacific Biosciences (PacBio) and Oxford Nanopore Technologies (ONT), as well as single-cell genome and transcriptome sequencing techniques.
Research Assistant Professor Chengxin Zhang, starting Spring 2023 in the Freddolino lab
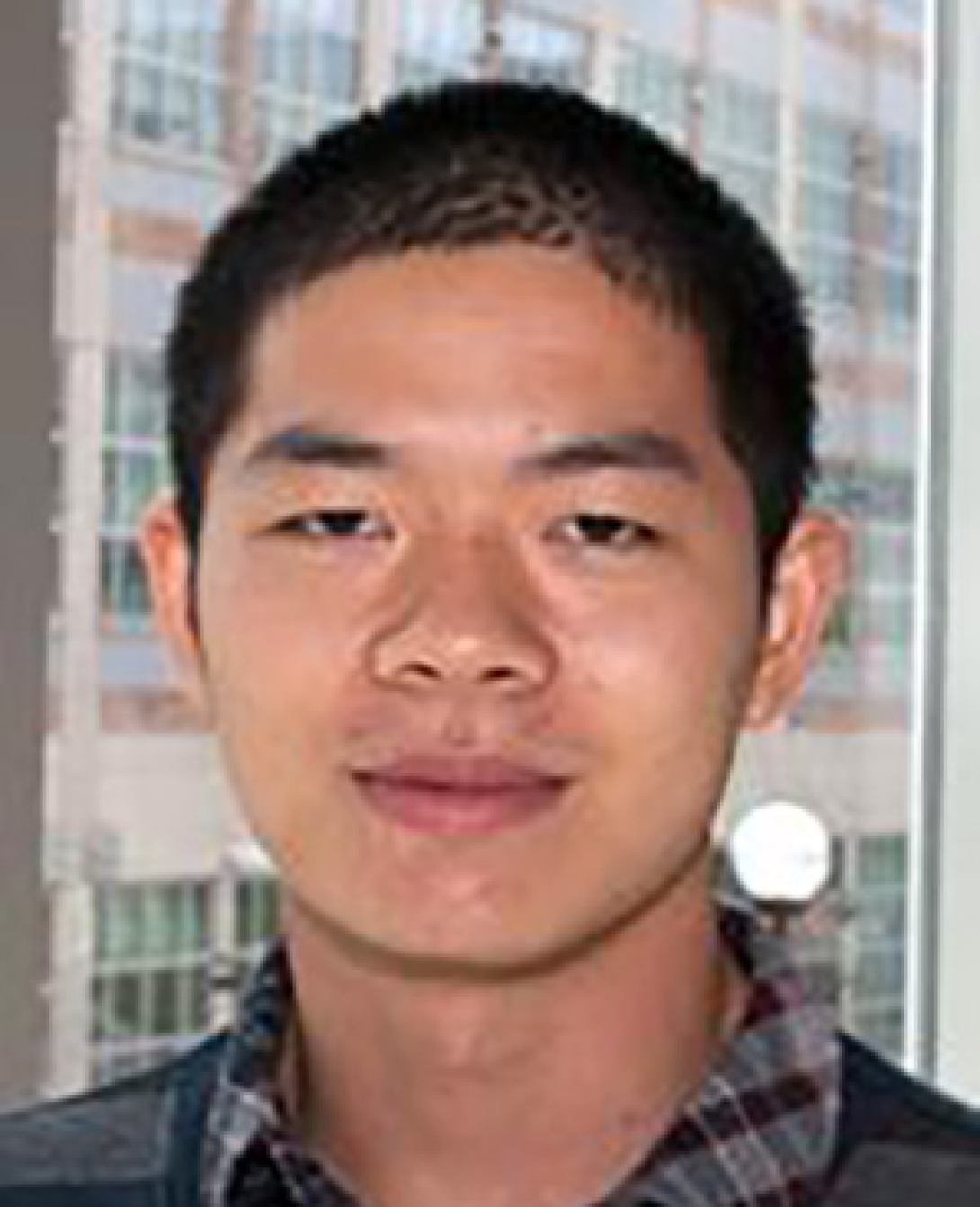
Zhang is interested in Algorithm development for protein and RNA structure prediction, function annotation, structure alignment and interaction prediction.
Research Assistant Professor Yue Zhao, starting Fall 2022 in the Sartor Lab
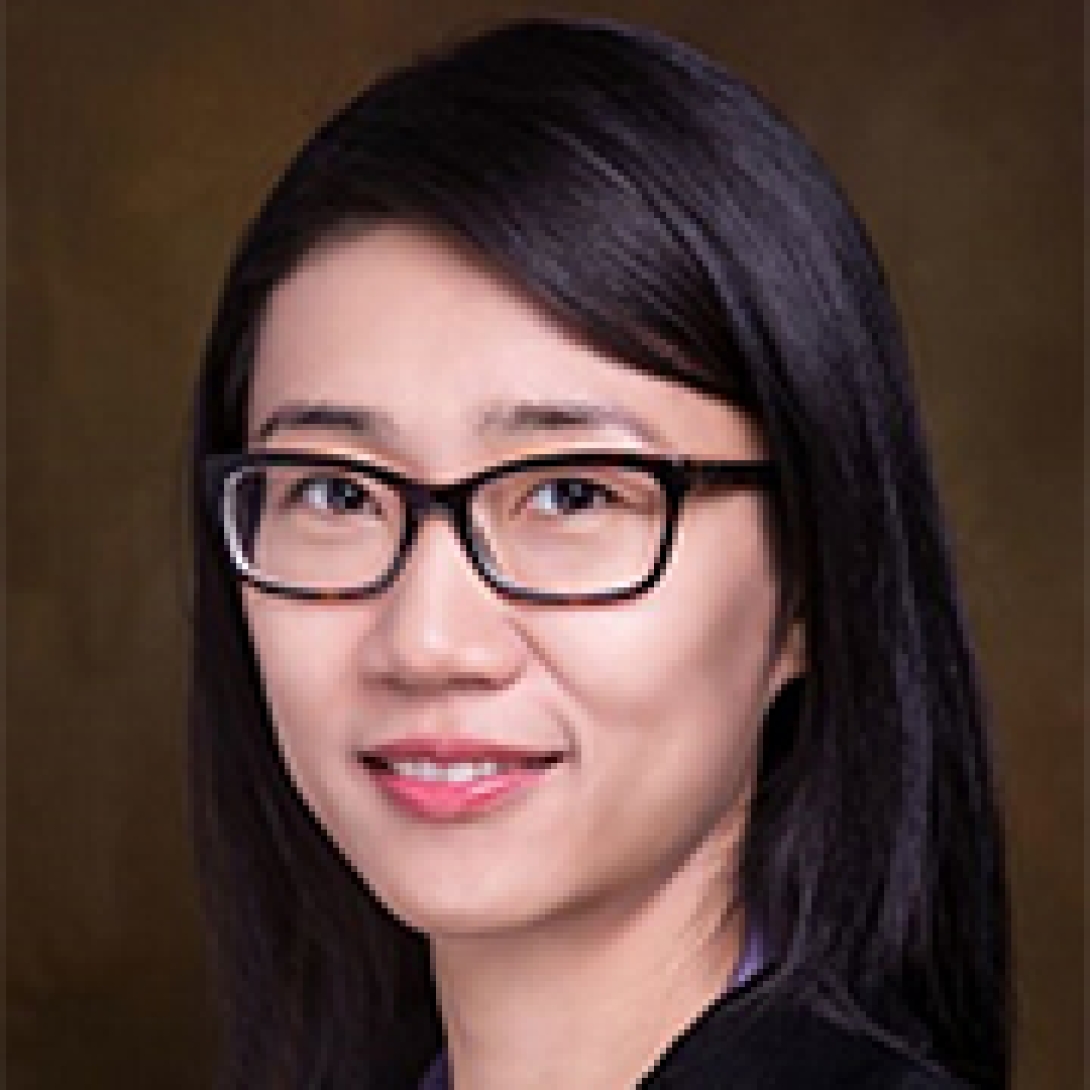
Dr. Zhao has tremendous experience working collaboratively with basic science researchers and physicians for designing optimal multi-omics data collection and performing sophisticated analyses, including but not limited to genomics, transcriptomics, proteomics, metabolomics, CRISPR-based genetic screening, and third generation sequencing.
To make meaningful contributions towards solving biomedical problems, Zhao is dedicated to delivering highly reliable predictions (e.g., predictive biomarkers and therapeutic target genes) by integrating all available data. In her most recent project, she developed a statistical method called CEDA (CRISPR screen with Expression Data Analysis) to integrate gene expression profiles with CRISPR pooled screen data to identify essential genes with higher abundance. CEDA pools the single guide RNAs (sgRNAs) targeting the genes with similar abundance to fit a hierarchical model, which can improve parameter estimation and reduce false positive rate significantly. CEDA is the first method that statistically integrates gene abundance into the target prediction model of genetic screen data. It lays a solid foundation for future development of integrative analysis methods for CRISPR screens.

Assistant Professor
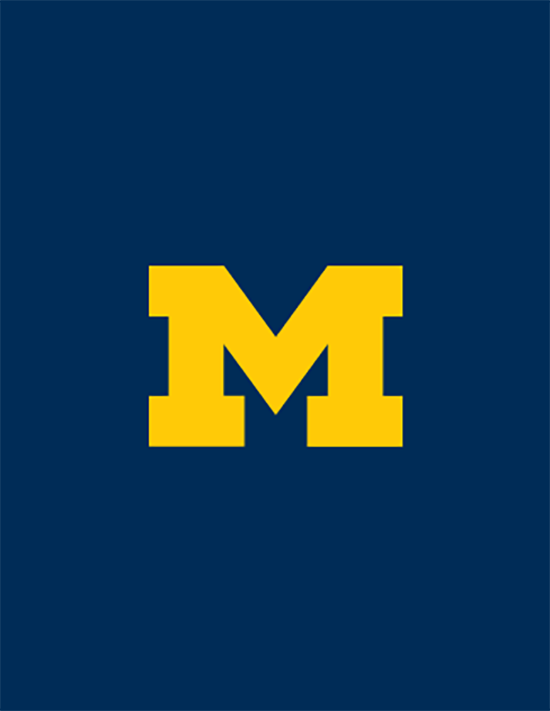
Research Assistant Professor

Assistant Professor

Assistant Professor

Professor
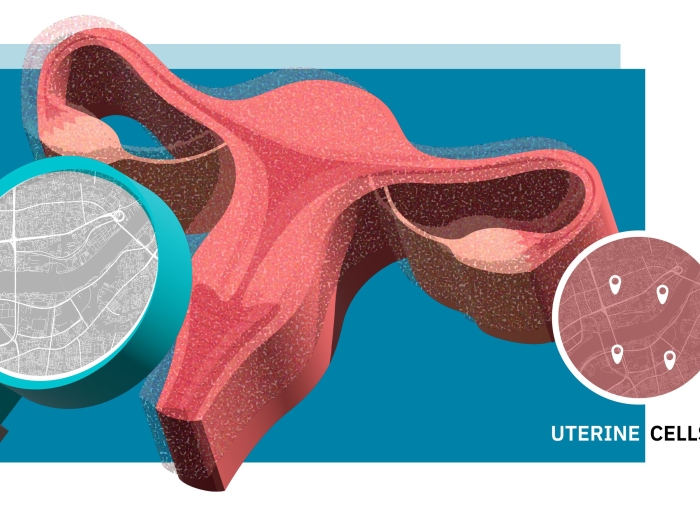



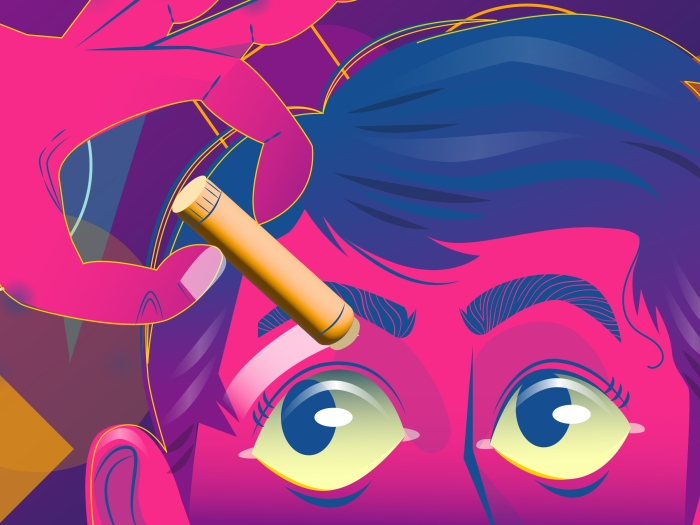
